|
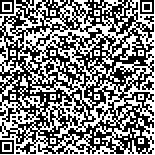 二维码(扫一下试试看!) |
基于多尺度特征融合注意力机制的纸病分类方法研究 |
Research on Paper Defect Classification Method Based on Multi-scale Feature Fusion Attention Mechanism |
收稿日期:2021-01-19 |
DOI:10.11980/j.issn.0254-508X.2021.04.004 |
关键词: 多尺度特征融合 注意力机制 卷积神经网络 图像分类 |
Key Words:multi-scale feature fusion attentional mechanisms convolutional neural network image classification |
基金项目:陕西省榆林市2020年科技计划项目(CXY-2020-090)。 |
|
摘要点击次数: 4664 |
全文下载次数: 2905 |
摘要:针对造纸工业中传统纸病分类算法准确率低的问题,本课题提出一种多尺度图像增强结合注意力机制的方法;采用锐化滤波器和对比度增强操作获得图像对直线信息的响应,同时利用Sobel边缘检测获取图像对边缘信息的响应,然后将这些响应分别放进卷积神经网络(CNN)中提取浅层局部信息后进行特征融合,得到全局信息,最后利用注意力机制,通过关注这些图像中最有特点的部分,进行纸病分类。实验表明,该方法优于HOG+SVM、LBP+SVM以及传统CNN等方法,在自建数据集上,分类准确率可达到96.63%;与现有基于CNN的纸病分类算法相比,所需的数据量更少,分类效果更好。 |
Abstract:Aiming at the problem of inaccurate classification of traditional paper defect Algorithm in paper industry, this study proposed a classification method combined with multi-scale feature fusion and attention mechanism. The method uses sharpening filter and contrast enhancement to obtain the response to straight line, and then used Sobel edge detection to obtain the response to edge. Then, the above responses were put into a convolutional neural network (CNN) separately to extract shallow local information and focuse to get global information, finally an attention mechanism was used to classify paper defect by focusing on the most characteristic parts of the image. Experiments showed that this method outperformed HOG+SVM, LBP+SVM and traditional CNN methods, achieving 96.63% classification accuracy on the self-built dataset. Compared with existing CNN-based paper defect classification algorithm, the method proposed in this study required less train data and obtained better results. |
查看全文 HTML 查看/发表评论 下载PDF阅读器 |