|
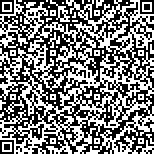 二维码(扫一下试试看!) |
基于PHAM-YOLO网络的卷烟纸燃烧线检测方法 |
PHAM-YOLO Network-based Detection Method of Combustion Line of Cigarette Paper |
收稿日期:2023-12-13 |
DOI:10.11980/j.issn.0254-508X.2024.03.016 |
关键词: 卷烟纸 燃烧线检测 YOLO 并行混合注意机制 |
Key Words:cigarette paper combustion line detection YOLO parallel hybrid attention mechanism |
基金项目:烟草行业标准项目“卷烟包灰性能测试方法”(2021B023);国家烟草专卖局重大科技项目“烟草行业质量监控大数据构建及应用研究”(110202101080(SJ-04))。 |
|
摘要点击次数: 1159 |
全文下载次数: 672 |
摘要:为实现卷烟纸燃烧时燃烧线的准确识别,构建了常见应用场景下的卷烟纸燃烧线数据集。针对检测背景复杂、多目标、燃烧线尺度不一且形状各异的难题,将并行混合注意机制嵌入了YOLO v5主干网络,构建了PHAM-YOLO网络模型用于卷烟纸燃烧线的检测。采用特征金字塔快速池化、边界盒回归等方法提升了卷烟纸燃烧线的定位准确性。结果表明,对于卷烟纸燃烧线数据集, PHAM-YOLO网络检测平均精度均值、精度和召回率分别为99.0%、99.8%和99.0%,其中平均精度均值比原始模型提高了5.0%,高于其他类型的目标检测方法。 |
Abstract:To determine the combustion line of cigarette paper, the dataset for cigarette combustion line detection was construct from common scenarios. To address the challenges of detecting complex backgrounds, multiple targets, varying scales, and shapes of combustion lines, a PHAM (parallel hybrid attention mechanism) was embedded into the YOLO v5 (you only look once, version 5) backbone network, and PHAM-YOLO was constructed for detecting multiple targets with varying scales and shapes in complex backgrounds. In addition, a spatial pyramid pooling fast (SPPF), the boundary box regression (BBR) module were introduced to improve the accuracy of combustion line positioning. The results showed that the proposed PHAM-YOLO network achieved the average precision mean (mAP), precision(P) and recall (R) of 99.0%, 99.8%, and 99.0%, respectively, where mAP was improved by 5.0% compared to the original model and higher than other types of target detection methods. |
查看全文 HTML 查看/发表评论 下载PDF阅读器 |