|
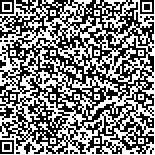 二维码(扫一下试试看!) |
基于DNN-LSTM的造纸废水处理过程温室气体排放分析模型 |
Analysis Model of Greenhouse Gas Emissions from Papermaking Wastewater Treatment Process Based on DNN-LSTM |
收稿日期:2023-10-01 |
DOI:10.11980/j.issn.0254-508X.2024.04.020 |
关键词: 深度神经网络 长短期记忆 造纸废水处理 温室气体 |
Key Words:deep neural network long short-term memory papermaking wastewater treatment greenhouse gas |
基金项目:国家自然科学基金(52000078)。 |
|
摘要点击次数: 988 |
全文下载次数: 5 |
摘要:本研究采用深度学习算法,对造纸废水处理过程的温室气体(GHG)排放进行建模与分析,以期为温室气体减排控制提供参考。结合造纸废水处理温室气体产生机理,在基准仿真1号模型(BSM1)的实验基础上,将深度神经网络(DNN)模型和长短期记忆网络(LSTM)模型用于造纸废水处理过程中温室气体排放建模与分析,以辅助温室气体在线监测和分析。结果表明,深度学习模型可以有效地捕捉温室气体排放的特征。模型验证结果R2>0.99,平均相对误差不超过1%。基于DNN的灵敏度分析结果表明,曝气强度、污泥排放量、溶解氧浓度及内循环流量是影响造纸废水处理过程温室气体排放的关键操纵变量,水质变量和操纵变量间的相互作用是影响温室气体排放的潜在因素。 |
Abstract:This study employed deep learning algorithms to model and analyze greenhouse gas(GHG) emissions in the papermaking wastewater treatment process, providing insights for GHG reduction and control. Based on simulation experiments on the basehine simulation model 1(BSM1), combined with the mechanisms of GHG generation in the papermaking wastewater treatment process, the deep neural network(DNN) and long short-term memory models (LSTM) were proposed for modeling and analyzing GHG emissions in the papermaking wastewater treatment process, to facilitate real-time monitoring and analysis of GHG. The results showed that deep learning could effectively describe GHG emission characteristics, with validation results showing R2 > 0.99 and average relative errors below 1%. The DNN model-based sensitivity analysis results showed that sludge discharge, dissolved oxygen concentration, and internal circulation flow rate were key manipulated variables influencing GHG emissions, while the interactions between water quality variables and manipulated variables constituted potential influencing factors for the GHG emissions. |
查看全文 HTML 查看/发表评论 下载PDF阅读器 |